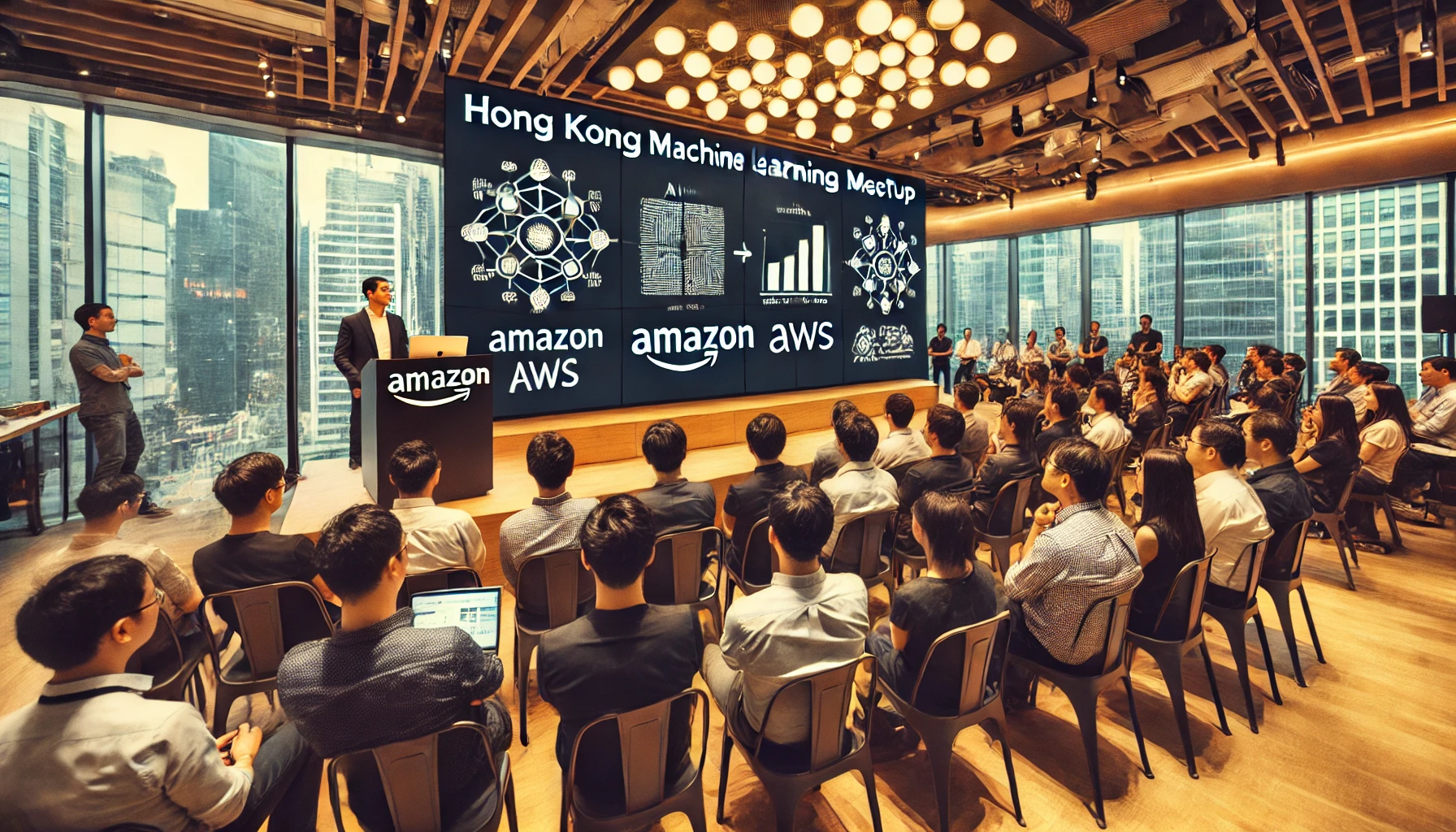
Hong Kong Machine Learning Season 6 Episode 6
04.10.2024 - Hong Kong Machine Learning - ~3 Minutes
When?
- Tuesday, December 10, 2024 from 6:00 PM to 9:00 PM (Hong Kong Time)
Where?
- This HKML Meetup is hosted at the AWS GenAI pop-up, Tower 535, in Hong Kong.
Thanks to Vahid Asghari (HKML) and Amy Wong (Amazon AWS) for helping making this event a success!
The page of the event on Meetup: HKML S6E6
Programme:
Talk 1: Responsible AI in the era of Generative AI
Abstract: Large Language Models (LLMs) have showcased remarkable proficiency in tackling Natural Language Processing (NLP) tasks efficiently, significantly reducing time-to-market compared to traditional NLP pipelines. However, upon deployment, LLM applications encounter challenges concerning hallucinations, safety, security, and interpretability. With many countries recently introducing guidelines on responsible AI application usage, it becomes imperative to comprehend the principles of constructing and deploying LLM applications responsibly. This hands-on session aims to delve into these critical concepts, offering insights into developing and deploying LLM models alongside implementing essential guardrails for their responsible usage.
Short bio: Bhaskarjit Sarmah is the Director and Head of RQA AI Lab at BlackRock, he applies his machine learning skills and domain knowledge to build innovative solutions for the world’s largest asset manager. He has have over 10 years of experience in data science, spanning multiple industries and domains such as retail, airlines, media, entertainment, and BFSI. At BlackRock, he is responsible for developing and deploying machine learning algorithms to enhance the liquidity risk analytics framework, identify price-making opportunities in the securities lending market, and create an early warning system using network science to detect regime change in markets. He also leverages his expertise in natural language processing and computer vision to extract insights from unstructured data sources and generate actionable reports. His mission is to use data and technology to empower investors and drive better financial outcomes.
Talk 2: Be helpful but don’t talk too much: Improving Multi-turn Emotional Support through Cognitive Principle of Relevance
Abstract: Cooprerative conversation is underpinned by multiple linguistic-pragmatic principles. The improvement demonstrates cogntive relevance as a rewarding goal for language models to achieve optimal relevance during communication, through maximizing cognitive effect while minimizing the processing effort imposed on the listener. To achieve and maximize user-prefered cognitive effect during interaction, Reinforcement Learning from Human Feedback (RLHF) has been widely adopted to empower LM-based conversation agents with the capability of producing positive cognitive effect. However, the minimization of user’s processing load, which is equally essential to cooperative conversation, has never been given sufficient attention. This study proposes a theory-driven reinforcement learning, Optimimal Relevance Learning (ORL), to improve the performance of language models in multi-turn emotional support conversation. The improvement demonstrates cogntive relevance as a rewarding goal for language models to acquire human-like communication ability.
Speaker: Justin Li is a third-year PhD student at the Department of Chinese and Bilingual Studies of The Hong Kong Polytechnic University. He is interested in conversation agent for social good, cognitive language modeling through eye-gaze data, and low resource NLP for Chinese and Arabic languages.