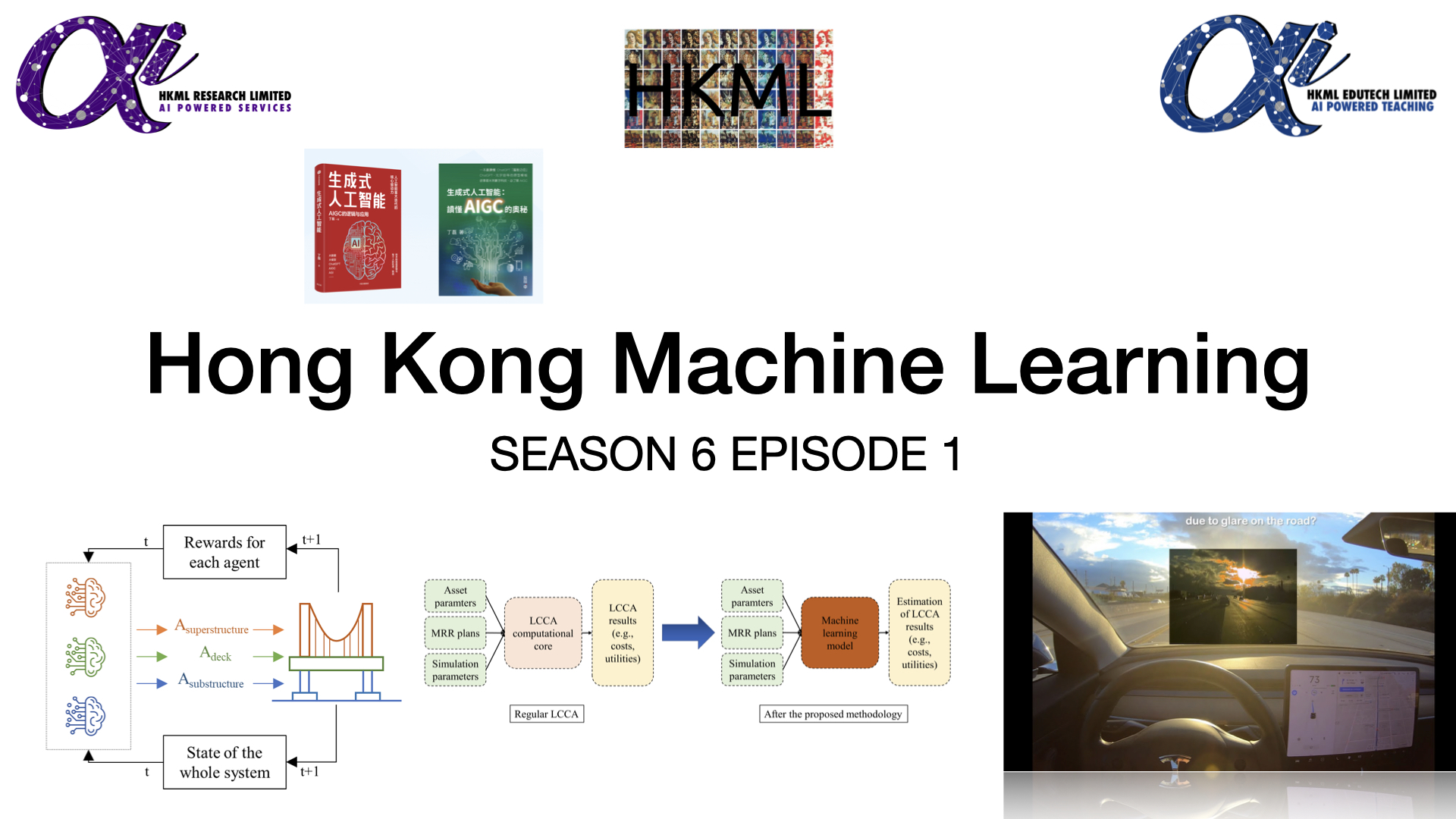
Hong Kong Machine Learning Season 6 Episode 1
29.11.2023 - Hong Kong Machine Learning - ~4 Minutes
When?
- Wednesday, November 29, 2023 from 7:00 PM to 9:00 PM (Hong Kong Time)
Where?
- This HKML Meetup is hosted at Hong Thai Expo & Business Center (also theDesk), 5/F, United Centre, 95 Queensway, Admiralty, Hong Kong.
Thanks to Vahid Asghari and Jiwei Li for helping making this event a success!
Networking event took place at the Oolaa Star Street bar.
The page of the event on Meetup: HKML S6E1
Programme:
Talk 1: ML for Infrastructure Management
Abstract: Infrastructure asset management tries to answer three questions: when, on which, and to what extent we should invest limited resources to maximize the benefits? Multiple non-linear uncertainties underlie this problem making it hard to solve. Academic solutions use accurate models but are computationally expensive; industry solutions use simplified models but are computationally feasible. Machine learning approaches can bridge this gap. In other words, ML can be used to accurately estimate the results of Monte Carlo simulation and non-linear optimization models in a negligible amount of time. Also, reinforcement learning can be used to replace the MC simulation and optimization for making desirable decisions. Finally, the application opportunities and challenges of these approaches in trading will be discussed in this presentation.
Speaker: Vahid Asghari is a quantitative trader focusing on the US equity market at Causality Group. His main academic and professional background focuses on financial decision-making using machine learning and statistical approaches. He spends most of his time developing new approaches and strategies for investment in various products in different markets. He has graduated with a PhD in engineering from HK PolyU, published more than 12 scientific papers, and mentored multiple students through their studies. Learning new tech skills, reading, and hiking are his main interests outside of work.
Talk 2: AI Landing in Business
Abstract: Despite significant progress in discriminative and generative AI in recent years, real business adoption of AI technologies is relatively slow. I will present a framework of leveraging AI models in business to generate lift in ROI. I will show cases in a variety of business domains, resulting in incremental revenue, reduced cost, or in some situations creating results that can be compared to Pareto optimality.
Speaker: Dr. Lei Ding is an experienced data science executive and consultant, and author of two popular books on business applications of AI. He has served Fortune 500 clients in finance, retail and manufacturing sectors. Serving as Chief Data Scientist, he led Data Science groups at PayPal and Baidu, responsible for a number of data systems that have brought in significant lift. Before, he led Data Science at Intent Media, an advertising startup in NYC. Earlier, he conducted applied research at Columbia University, UIUC, and IBM Watson in the areas of large-scale machine learning, artificial intelligence, and computer vision. He studied management at Stanford.
Talk 3: Helping robots to help humans
Abstract: In the realm of robotics and deep learning, two significant challenges are object recognition under varying lighting conditions and successful object grasping. A solution for each of these problems is presented. The first piece of research addresses the former by proposing a novel approach using Generative Adversarial Networks (GANs) to normalise the illumination of an input image, thereby enhancing object recognition performance. This method outperforms traditional techniques that rely on contrast normalisation or large amounts of annotated data, demonstrating its effectiveness and scalability. Building on this foundation of improved object recognition, the second research work focuses on the crucial aspect of object grasping. Instead of computing the full 6D pose for the object of interest or predicting a set of grasping points, both of which have limitations in scalability and generalisation, the authors propose a method that teaches a robot how to grasp an object through a short human demonstration. This approach involves using a sequence of RGB-D images to build associated hand and object meshes, completing missing parts of the reconstructed object shape, and transferring the relative pose between the object and human hand into grasping instructions for the robot.
Speaker: Luca Minciullo is an AI scientist, currently working for LifeHikes. With over half a decade of industry R&D experience in AI, his expertise spans diverse fields such as medical imaging, computer vision for indoor robotics, and mobile phone applications. Luca holds a PhD in Computer Science from The University of Manchester. Prior to this, he completed his Master’s and Bachelor’s degrees from La Sapienza University of Rome, the city where he was born and spent his formative years. He has made significant contributions to the field of AI, with his work being published in prestigious conferences and journals including ICCV, WACV, and PAMI. Additionally, he holds over 10 patents across Europe, Hong Kong, and Mainland China.