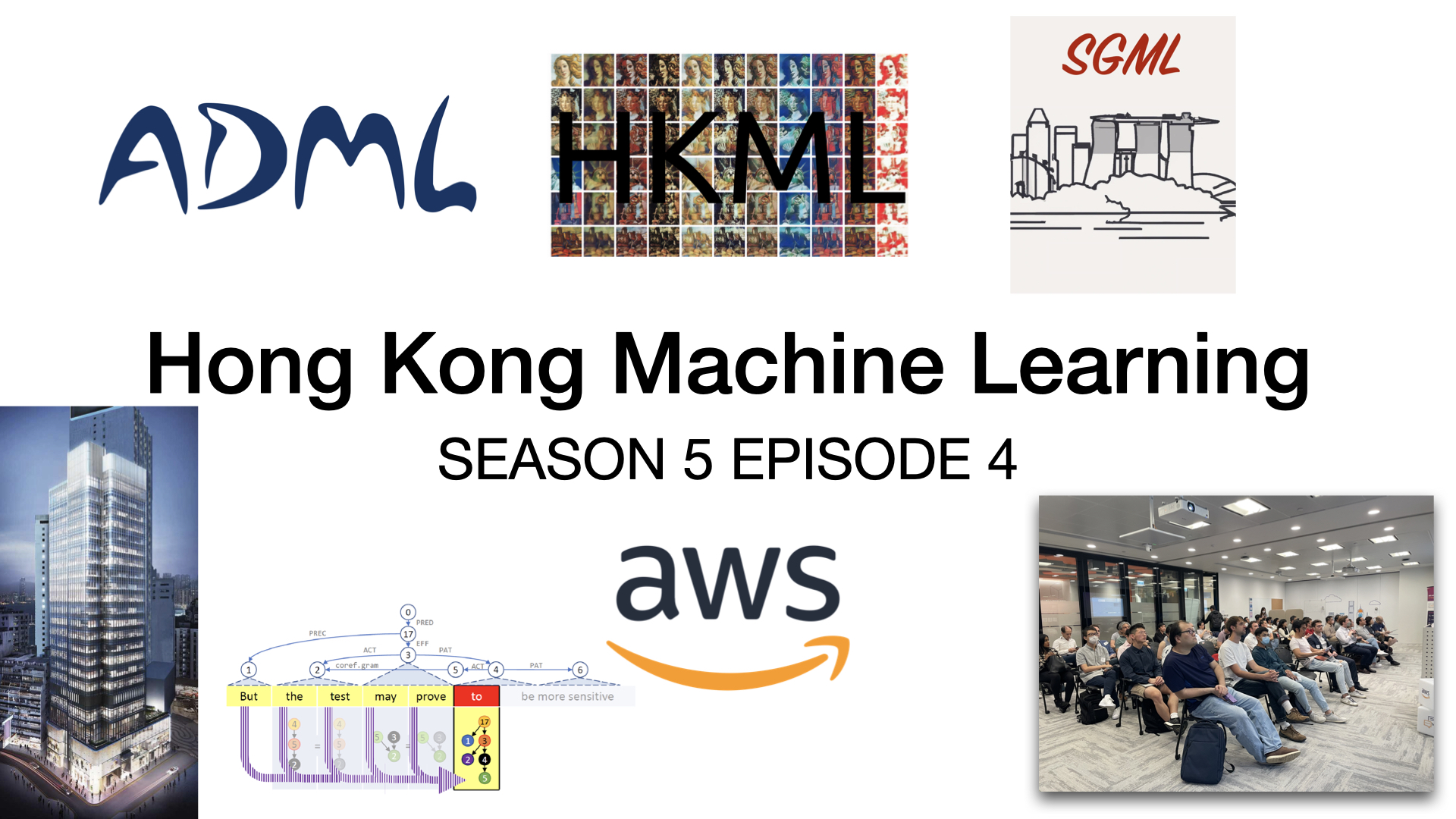
Hong Kong Machine Learning Season 5 Episode 4
11.05.2023 - Hong Kong Machine Learning - ~4 Minutes
When?
- Thursday, May 11, 2023 from 6:30 PM to 8:30 PM (Hong Kong Time)
Where?
- This HKML Meetup is hosted at Amazon AWS office (Tower 535) in Causeway Bay, Hong Kong.
Thanks to Amy Wong, Andrea Cheung, Sam and Christy for helping making this event a success!
Networking event took place at the ALTO rooftop bar.
The page of the event on Meetup: HKML S5E4
Programme:
Talk 1: Large Language Model at AWS - Generative AI and BloombergGPT sharing
Abstract: Generative AI is a type of AI that can create new content and ideas, including conversations, stories, images, videos, and music. It is powered by large models that are pre-trained on vast amounts of data and commonly referred to as foundation models (FMs). With generative AI on AWS, you can reinvent your applications, create entirely new customer experiences, drive unprecedented levels of productivity, and transform your business. You can choose from a range of popular FMs, or use AWS services that have generative AI built in, all running on the most cost-effective cloud infrastructure for generative AI.
Speaker: Yanwei Cui, PhD is the Machine Learning Specialist Solutions Architect at AWS. He started machine learning research at IRISA (Research Institute of Computer Science and Random Systems), and has several years of experience building artificial intelligence powered industrial applications in computer vision, natural language processing and online user behavior prediction. At AWS, he shares the domain expertise and helps customers to unlock business potentials, and to drive actionable outcomes with machine learning at scale. Outside of work, he enjoys reading and traveling.
Talk 2: Measuring the Impact of Remote Work Across the United States
Abstract: Discover how a framework using novel data and machine learning can measure remote work at the firm-level. See the impact of remote work on employee internet activity and how it can affect a county’s mobile phone data on workplace visits. Explore the determinants and consequences of remote work with cross-sectional variation.
Speaker: Dr. Alan Kwan, tenure-track faculty member at the University of Hong Kong, specializes in applying alternative data for insights in corporate finance, asset management, and investing. His work has been recognized by top journals and conferences across finance, economics, and science, including the American Economic Review and Science Advances. Dr. Kwan graduated from Dartmouth College and received his PhD from Cornell in Finance. He is also a team member of asset management firm Chicago Global Services, based in Singapore.
Talk 3: Can a pretrained neural language model still benefit from linguistic symbol structure? Some upper and some lower bounds
Abstract: Explore how deep-learning-based language models and symbolic linguistics can be reconciled through simple vector encoding of labeled and unlabeled linguistic structure. Discover how different linguistic representations perform on next-word prediction tasks and their robustness against noise. Learn about the potential for drastic improvements to language model perplexity with human-like linguistic knowledge resources and the challenges of automatic parsers.
This is joint work with Emmanuele Chersoni, Nathan Schneider, and Lingpeng Kong.
Speaker: Jakob Prange is a postdoctoral fellow at the Department of Chinese and Bilingual Studies at Hong Kong PolyU. He holds a PhD in Computer Science and Cognitive Science from Georgetown University and an undergraduate degree in Computational Linguistics from Saarbruecken, Germany. Jakob’s research focuses on designing and improving linguistic representations for machine learning techniques, while also considering foundational linguistic perspectives.
Talk 4: Comparing and Predicting Eye-tracking Data of Cantonese and Mandarin
Abstract: This paper introduces the first deeply-annotated joint Mandarin-Cantonese eye-tracking dataset, from which we achieve a unified eye-tracking prediction system for both language varieties. In addition to the commonly studied first fixation duration and the total fixation duration, this dataset also includes the second fixation duration which is thought to reflect later and structural processing. A basic comparison of the features and measurements in our dataset revealed variation between Mandarin and Cantonese on fixation patterns related to word class and word position. The test of feature usefulness suggested that traditional features are less powerful in predicting structural processing, to which the linear distance to root makes a leading contribution in Mandarin. In contrast, Cantonese eye-movement behavior relies more on word position and part of speech.
Speaker: Junlin Li is a postgraduate student at the department of Chinese and Bilingual Studies of PolyU. He is interested in low-resource NLP, cognitive processing, and its application in NLP.