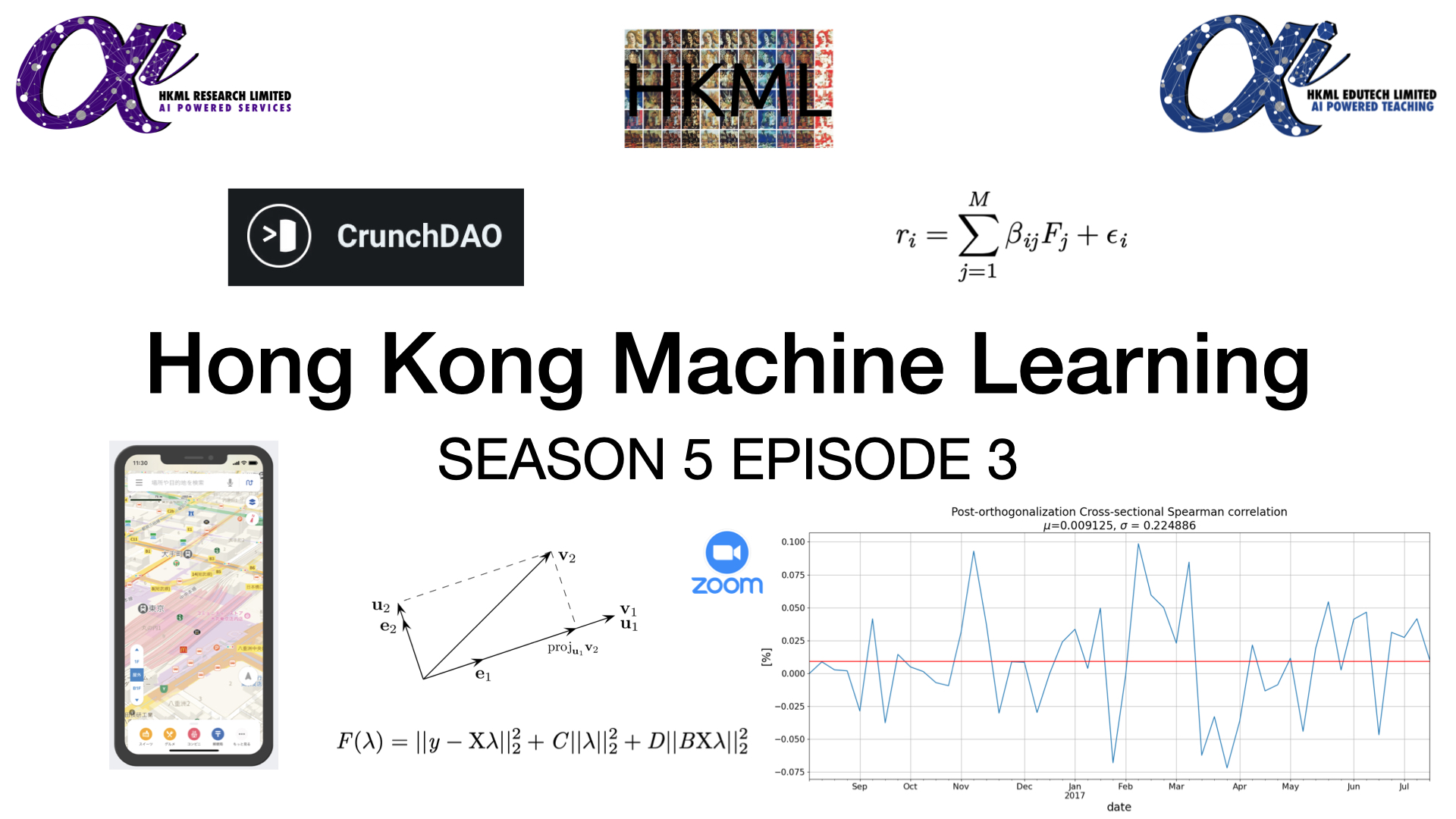
Hong Kong Machine Learning Season 5 Episode 3
23.02.2023 - Hong Kong Machine Learning - ~3 Minutes
When?
- Thursday, February 23, 2023 from 7:00 PM to 9:00 PM (Hong Kong Time)
Where?
- This meetup was hosted online on Zoom.
The page of the event on Meetup: HKML S5E3
Programme:
Talk 1: Nonlinearities in a multi-factor model framework using Machine Learning
Speaker:
Matteo is the lead quant researcher of CrunchDAO. With a background in machine learning and dynamical systems theory. He graduated in Space Flight with a Talent Scholarship from TU Delft, worked as a researcher in the Horizon2020 program by the European Commission and worked as a flight dynamics software engineer for the European Space Agency. Together with working in quantitative finance, he is one of the developers of CrunchDeSci, a Decentralized Science platform used to perform research in a reproducible manner.
Abstract:
CrunchDAO’s Crowdsourced Investment Framework makes use of supervised learning to predict returns, which are residualized against, i.e., uncorrelated with linear econometric risk models. In order to obtain estimates with the desired properties, in this work we investigate the effect of design choices associated with feature engineering and model training. In particular, the orthogonality condition between factors and estimations can be imposed indirectly training on an orthogonal feature space. Alternatively, (non-linear) machine learning models can be trained, imposing orthogonalization in the definition of the fitness function. We show the consequences, in terms of out-of-sample accuracy, of these design choices, both for a feature space orthogonal and not orthogonal to risk factors. In the context of crowdsourced investment research through tournaments, we explore the potential of confidential computing to align the interests of tournament players with that of portfolio managers; from a game theory, perspective, we discuss the need to be able to define a Nash equilibrium in the CrunchDAO tournament.
Talk 2: Applied/Production ML/RL for Ad/Recommendation
Speaker:
Kevin Noel, Lead of Ad Machine Learning at Mapbox Japan. Kevin focuses on real time Geo-Spatial Ad targeting/Recommendation. Previous experience includes Principal in Data Science/Recommendation at Big Data Tech Company (Japan) and in financial industry (quantitative fields at Big Banks).
Abstract:
Reinforcement Learning has recently gained popularity with the successes in solving difficult high dimensional problems in games, optimization. However, RL is not limited to game-like problem and covers different range of application as well as a broad science field (from ML theory to Optimal control). So, we highlight key principles of RL and challenges of applying ML/RL for real life/production level for Ads/Recommender. Particularly, we illustrate through a sub-field of RL, called Stochastic Bandit, and his variants, tailored for low latency and high volume inference, typical in online Big Data field. In addition, we will mention active areas of applied research related to online Ads/Rec such as offline policy methods, causal methods to improve robustness, evaluation.
We highlight processes to productionize Reco algo Ad Optimization, in Japanese context. On the one hand, we describe specificities of Ad data, as well as part of Geo-Spatial data. On other hand, we illustrate the technical challenges with Online RL/Bandit, from Pytorch side to various data pipelines requirements, different from standard ML. Finally, we summarize the takeovers and highlight the specificities of Japan for ML/Data environment with example from Local Industry.
Video Recording of the HKML Meetup on YouTube
- YouTube videos:
HKML S5E3 - Nonlinearities in a multi-factor model framework using Machine Learning by CrunchDAO
HKML S5E3 - Applied/Production ML/RL for Ads/Recommendations