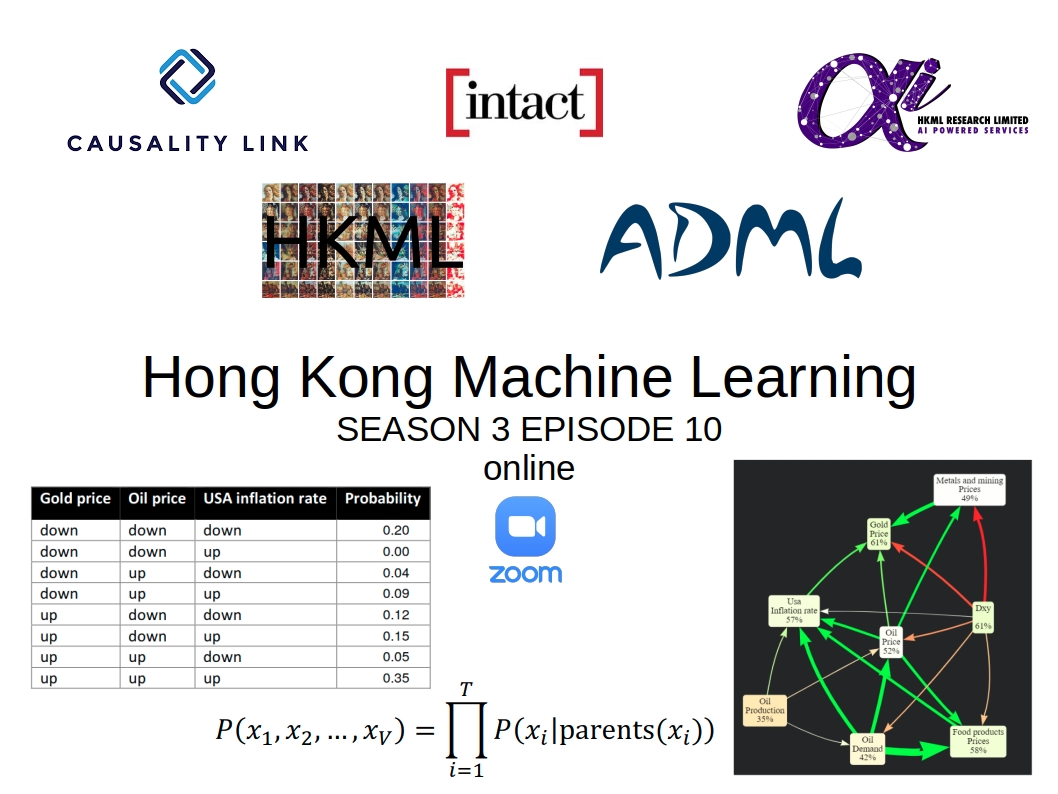
Hong Kong Machine Learning Season 3 Episode 10
05.05.2021 - Hong Kong Machine Learning - ~3 Minutes
When?
- Wednesday, May 5, 2021 from 7:00 PM to 9:00 PM (Hong Kong Time)
Where?
- At your home, on zoom. All meetups will be online as long as this COVID-19 crisis is not over.
Programme:
Talk 1: Building Probabilistic Causal Models using Collective Intelligence
Alexander Denev, Head of AI at Deloitte, Lecturer at Oxford. Author of:
-
Book of Alternative Data: A Guide for Investors, Traders and Risk Managers
-
Probabilistic Graphical Models: A New Way of Thinking in Financial Modelling (cf. my summary of this book on this blog)
-
Portfolio Management under Stress: A Bayesian-Net Approach to Coherent Asset Allocation
Olav Laudy, Chief Data Scientist, Causality Link
Abstract:
The purpose of this paper is to show a novel approach to automatically generating Probabilistic Causal Models (Bayesian Networks (BN)) by applying Natural Language Processing (NLP) techniques to a corpus of millions of digitally published news articles in which views by different authors are expressed on the future states of economic and financial variables, and geopolitical events. The BNs that we will show how to derive will represent the wisdom-of-the-crowds forward-looking point-in-time views on various variables of interest and their dependencies. These Bayesian Networks are likely to be of interest to asset managers and to economists who want to gain a better understanding of the current drivers of an economy based upon a rigorous probabilistic methodology. Additionally, in an asset allocation context, the BNs we derive can be fed to an optimization engine to construct a forward-looking optimal portfolio given the constraints of the asset manager (e.g., budget, short constraints etc.). We demonstrate various automatically derived Bayesian Networks in a financial context.
Keywords: NLP, causal graphs, Bayesian Networks, macro-finance, expert elicitation, knowledge mining.
paper: https://papers.ssrn.com/sol3/papers.cfm?abstract_id=3808233
You can find the slides of the talk there.
Talk 2: Case Study: Intact lab’s learning after 1 year in ML in investment
Kedi Wang and Sam Cheung from Intact Financial (HK) Limited (Intact Lab).
This presentation will start with a quick introduction on who Intact is & motivation/context of the project, then it will talk about some of the technical challenges faced by the team and discuss many of the learning from the last year. This presentation’s technical portion focus more on data pre-processing challenges, solutions used to solve our pragmatic challenges in the project, and touches on some of the classical ML algorithms experimented with.
Video Recording of the HKML Meetup on YouTube
- YouTube videos:
HKML S3E10 - Building Probabilistic Causal Models using Collective Intelligence
Thanks to our patrons for supporting the meetup!
Check the patreon page to join the current list: