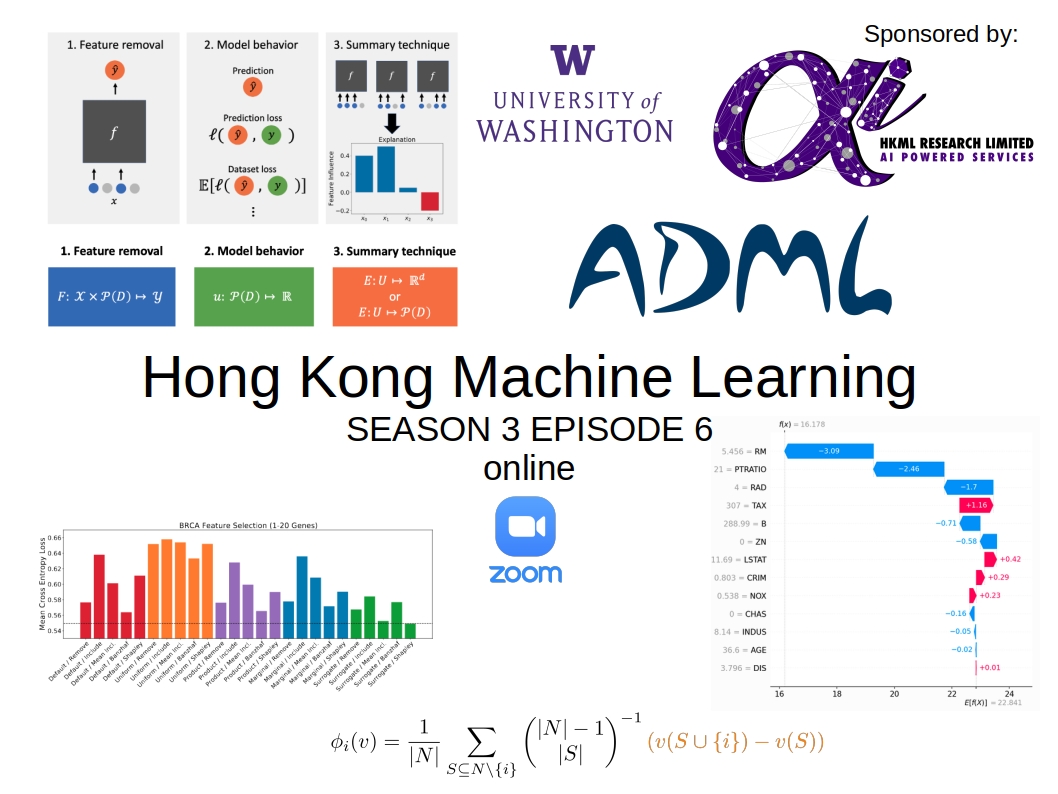
Hong Kong Machine Learning Season 3 Episode 6
12.01.2021 - Hong Kong Machine Learning - ~3 Minutes
When?
- Tuesday, January 12, 2021 from 9:00 PM to 11:00 PM (Hong Kong Time)
Where?
- At your home, on zoom. All meetups will be online as long as this COVID-19 crisis is not over.
Programme:
Talk 1 (chaired by Gautier Marti): Feature Selection and Importance by Maxence de Rochechouart
-Short Bio
After Maxence completed Mathematics studies (BSc + MSc) in England, he pursued with the Master MVA from ENS Cachan in France. This specialised degree in Machine Learning/Computer Vision offers a unique exposure to the field of Machine Learning, and the related research, with some key researchers from the public as well as private sector among the teaching staff. After six months of classes, Maxence completed this degree with a six month end of studies internship at BNP Paribas, where he was working on supply-chain data.
-Abstract
When extracting information/making predictions from a large dataset a key step, before choosing and tuning a model, is feature selection. As opposed to including all predictors in the model, a wise variable selection will dramatically reduce training time and improve the performance of the model. In some cases, it can also help making the predictions more interpretable. After presenting some commonly used ways to select features before choosing a model, I will present feature selection and importance within the framework of random forests, where the model and feature importance are built simultaneously. I will discuss different methods depending on the nature of the task to be accomplished with the data; for example, to interpret the information from a dataset on a target variable, as many significant features as possible should be included, whereas for prediction tasks, the number of features should be minimised while maximising the performance of the model.
Talk 2 (chaired by Gautier): Explaining by Removing: A Unified Framework for Model Explanation by Ian Covert
Ian Covert presents his paper on feature importance and model explanation in machine learning. More precisely, he introduces a mathematical framework to unify several seemingly different methods (e.g. LIME, SHAP) under the ‘removal-based’ concept.
Before this paper you had to understand 25+ seemingly very different feature importance methods, but now you just need to understand that you can combine 3 different choices of functions to cover the space of removal-based explanations.
Paper: https://arxiv.org/pdf/2011.14878.pdf
The paper is 80 pages. For an extractive summary: Check this blog.
Video Recording of the HKML Meetup on YouTube
- YouTube videos:
HKML S3E6 - Feature Selection and Importance by Maxence de Rochechouart
HKML S3E6 - Explaining by Removing: A Unified Framework for Model Explanation by Ian Covert
Thanks to our patrons for supporting the meetup!
Check the patreon page to join the current list: