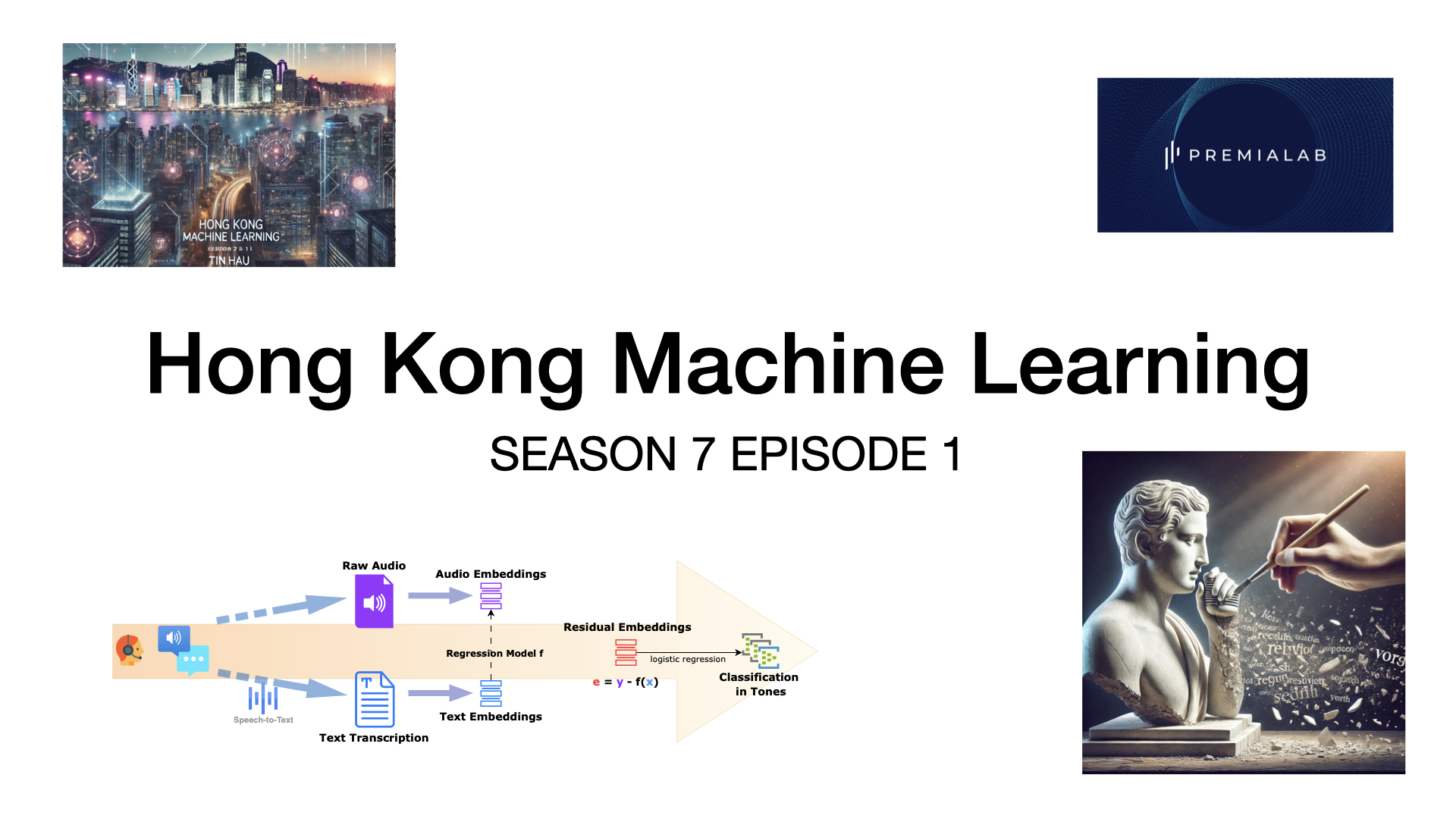
Hong Kong Machine Learning Season 7 Episode 1
20.02.2025 - Hong Kong Machine Learning - ~2 Minutes
When?
- Thursday, February 20, 2025 from 6:30 PM to 8:30 PM (Hong Kong Time)
Where?
- This HKML Meetup is hosted at Premialab, Tin Hau, in Hong Kong.
Thanks to Pierre Trecourt (COO Premialab), Vahid Asghari (Premialab) and Kevin Couton (Premialab’s Head of Software Engineering) for sponsoring this event and helping making it a success!
The page of the event on Meetup: HKML S7E1
Programme:
Talk 1: Teaching Machines to Hear the Unsaid - Extracting Tone from Speech Embeddings
Abstract: Self-supervised learning models for speech processing, such as wav2vec2, HuBERT, WavLM, and Whisper, generate embeddings that capture both linguistic and paralinguistic information, making it challenging to analyze tone independently of spoken content. In this work, we introduce a method for disentangling paralinguistic features from linguistic content by regressing speech embeddings onto their corresponding text embeddings and using the residuals as a representation of vocal tone. We evaluate this approach across multiple self-supervised speech embeddings, demonstrating that residual embeddings significantly improve tone classification performance compared to raw speech embeddings. Our results show that this method enhances linear separability, enabling improved classification even with simple models such as logistic regression. Visualization of the residual embeddings further confirms the successful removal of linguistic information while preserving tone-related features. These findings highlight the potential of residual embeddings for applications in sentiment analysis, speaker characterization, and paralinguistic speech processing.
Short bio: Gautier Marti is a quant trader and researcher, focusing on finding alphas for systematic strategies typically implemented in sophisticated quant hedge funds.
Material:
- Disentangling Speech Embeddings: Removing Text Content from Audio Embeddings with Regression
- Serious, Sassy, or Sad? Teaching Machines to Read the Room (From Speech Embeddings)
Talk 2: Training LLMs for text-adventure games
Abstract: Large Language Models (LLMs) have become essential components in various autonomous agent systems. Recent advancements leverage fine-tuning with expert trajectories to improve agent performance in complex decision-making tasks. This talk will explore recent fine-tuning algorithms for LLMs, providing a comparative analysis of several state-of-the-art methods. The discussion will be grounded in empirical evaluations of standard text-adventure game datasets, highlighting key insights, and future research directions.
Short bio: Chung Wang Wong is an AI quant researcher at Qube Research & Technologies